IOUG Podcast 10-AUG-2012: The Big Data World of the Data Scientist & The DBA
-
Posted by Harry E Fowler
- Last updated 6/25/19
- Share
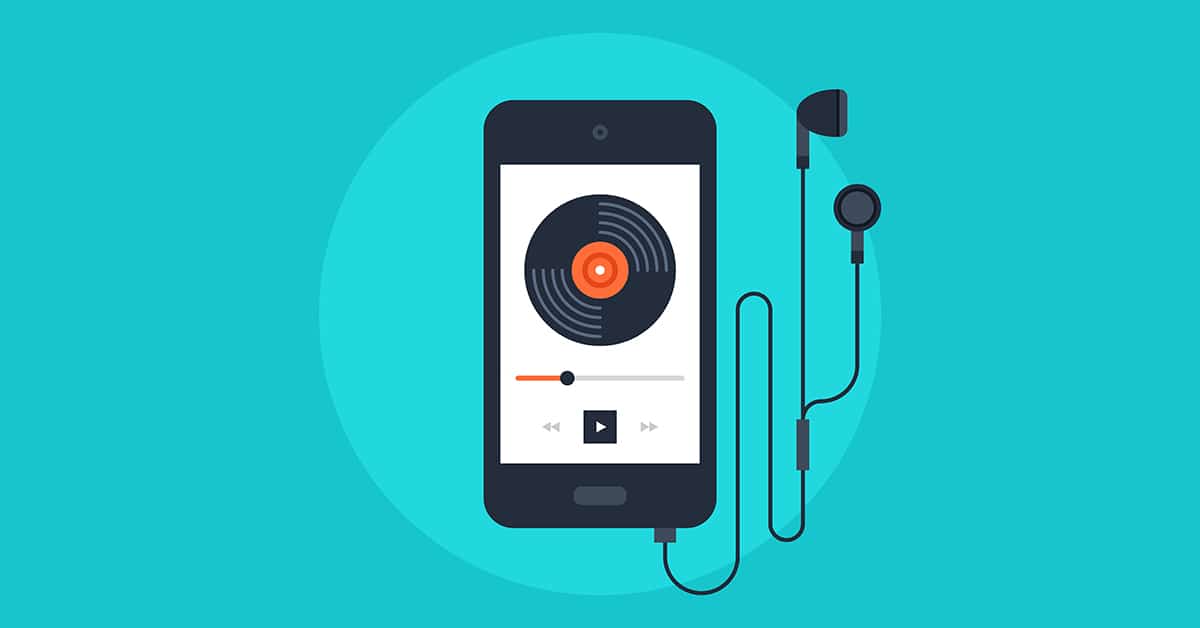
For the week of August 10th, 2012:
- So, Just What is a Data Scientist
- Big Data at Work
- The DBA Evolution
“IOUG Podcast 10-AUG-2012: The Big Data World of the Data Scientist & The DBA”
Subscribe to this Podcast (RSS) or iTunes
**Please note in this particular podcast there are numerous links to online references that can be found by reviewing the transcript of this episode at our website blogs.ioug.org
What Is A Data Scientist
During this year’s IOUG Leadership Summit we discussed the evolving role of two key new roles, that of the DBA Operator (known for many years as a Production DBA – the one responsible for ”keeping the lights on” but usually cannot alter program code or make modifications to existing structures), and the emergence of the modern Data Scientist.
40 years ago when John Tukey, a pioneering statistician, coded the PRIM-9 program (Picturing, Rotation, Isolation, and Masking of data in up to 9 dimensions) on an IBM 360/91, the data scientist was a statistician good at working with computers to figure out how data populations sorted according to the familiar bell curve of normal distributions. As computing power increased, the ability to generate more than statistical inferences about data emerged, and in 2001 William Cleveland coined the term “data science” referring to the analysis of patterns and trends found by looking at the various groups and re-combinations of various statistical results.
DJ Patil, a scientist at LinkedIn back in 2009, when the company was first emerging in the infantile social media space, thought about how the whole field was missing a simple job description, comparing data engineers to how the character of Mr. Spock (the Science Officer) often was left out of tactical decision-making on Star Trek: TOS episodes. Patil decided that the term “Data Scientist” could mean something broader when other job descriptors such as “analyst”, “research technician”, and “business intelligence” didn’t properly define the emerging role of taking petabytes worth of data and developing inferential explanations of what the data really meant, as opposed to simply measuring statistical endpoints within large data sets. But those kinds of Data Scientists simply didn’t exist yet. And thus organizations like Kaggle were created to collect and focus like-minded individuals with the capacity to deal with huge data on a solution-seeking basis.
Cooperatives, such as Kaggle, emerged by specializing in conducting predictive modeling competitions. Companies, governments, and researchers present data sets and problems which are matched with the world’s best data scientists who then compete to produce the best solutions. At the end of a competition, the competition host pays prize money in exchange for the intellectual property behind the winning model. This form of intellectual interaction normalizes the playfield between the data science wunderkinds and the highly-credentialed post-doctoral engineers whom all vie for the right to claim the title of “best data solution.”
Big Data at Work
O’Reilly Publishing in its “Big Data Now” primer on such topics has author, Mike Loukides defining the term “data science” for us. As the world is filled with data-driven applications, having a database and middleware to transform and present it to us, it is the value of the data itself, and the byproduct data created from the evaluation process that becomes the “science” in Data Science. Once the data provides a solution set, it becomes transformed into a data product or a set of information with intrinsic value beyond the original data upon which it is based.
An article in this month’s Wired UK magazine, “The Exabyte Revolution”, authored by Neal Pollack explores many different dimensions of this new role in significant depth, so we thought we’d bring you this week the highlights of some of the real-world examples of how the Data Scientist’s role is evolving.
Some Examples of Big Data and Data Scientists at Work:
Gracenote, the music and sound media indexing giant, started with collecting data on every published piece of music in the world and created hashing algorithms to uniquely identify every piece of published sound creating primary keys in the process. Those key lookups became the way modern music players can display the artist, album art, and liner notes of every piece of music in the world. And now they’ve added more metadata to interpret the media to personalize your experience as a listener and viewer in it’s new Habu data product by analyzing similarities in the types of music you listen to, when you’re listening to it, and even where, such as, in a car, at home, or while walking around. All that data gets transformed into mood algorithms that can present alternative media based upon your listening habits (similar to the iTunes Genius model but even smarter).
The Global Viral Forecasting Initiative (GVFI), founded by Dr. Nathan Wolfe, gathers data from multiple sources, including viral discovery in the field, anthropological research, over-the-counter drug sales, and social media trends, to predict and prevent outbreaks and helping to track animal-borne viruses.
The Santa Clara Police Department (California) created an international data warehouse and reporting analysis system (publically accessible at CrimeReports.com) to accumulate data from all crime records from world-wide enforcement agencies. Then analyzes the data using predictive algorithms to generate daily crime potential maps, and high-risk time window alerts resulting in a 27 percent reduction in overall crime incidents since 2010.
Netflix leveraged the Kaggle network by using its collection over 100 million customer media ratings and awarded a prize of $1 million to the team who could improve its prediction of user ratings for its film selections by at least 10 percent. Algorithms like this result in those amazing “We thought you might also like…” side-title suggestions now commonly found on every e-commerce site in the world.
Google Books, the massive online electronic library initiative, now spanning over 5.2 million books, created the Ngram Viewer (books.google.com/ngrams) which can analyze the usage of words and phrases from the past 6 centuries and show relationships in lexicographical evolution from every published work. This engine allows users to understand when words first emerged and when they became obsolete – and more interestingly, what new words were derived from those in the past and what significant events accompanied the transition.
Wal-Mart created an intelligent inventory and supply-chain management system that analyzes demand patterns and sales trends by geographic region and other demographic slices, allowing more efficient distribution and unit spread of its available stock, reducing the frequency of out-of-stock and over-stock conditions throughout its network of retail stores and websites.
The DBA Evolution
Database Administrators (DBAs) have often been the solution stopping-point for most functional new demands within enterprises because they, by responsibility of their own job function, end up understanding where data resides and who are the producers and consumers of that data. When storage administrators want to know why disk space is being rapidly consumed, the DBAs track down which process is creating it and determine whether the volume is within normal ranges or deviant. When architects are designing data flows and infrastructure requirements, the DBAs are at the front-line of figuring out what instances are connected, and what their future growth projections might be. Taking that knowledge and understanding one step further and leveraging experience in data structure design, keeping up with the latest database technologies, and understanding holistic tuning practices becomes the natural progression into the Data Scientist role. After all, as often as data scientists have often been labeled with possessing “magical powers” by divining solutions from massive data sets, so too, have DBAs been dubbed as having a “magic” touch when it comes to recovering dead databases, tuning the impossible, and increasing throughput by tweaking mystical parameter changes and often using upside-down logic. The DBAs design, build and manage the buildings that our data lives in and it makes sense they might have a good idea of how a million of those buildings have transformed into a city or two.